The propagation of coherent light through a thick layer of scattering material is an extremely complex physical process. However, it remains linear, and under certain conditions, if the incoming beam is spatially modulated to encode some data, the output as measured on a sensor can be modeled as a random projection of the input, i.e. its multiplication by an iid random matrix. One can leverage this principle for compressive imaging, and more generally for any data processing pipeline involving large-scale random projections. This talk will discuss recent technological developments of optical co-processors within the startup LightOn, and present a series of proof of concept experiments in machine learning, such as transfer learning, change point detection, or recommender systems. |
Laurent Daudet
Optical random features for large-scale machine learning
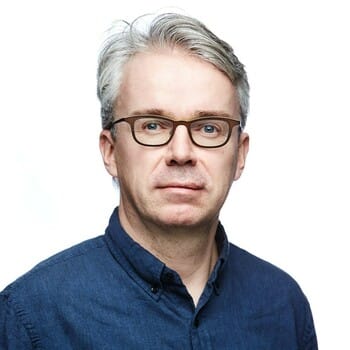
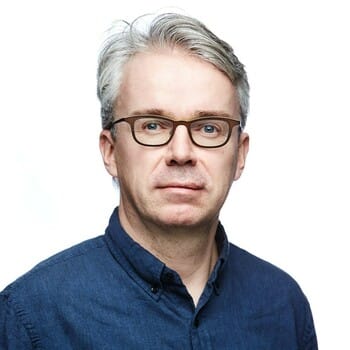
Date & heure
27/03/2019
Lieu
ENS – 24, rue Lhomond – 75005 Paris, Room : IV
Accueil