Aaron Tranter
Deep learning cold atomic ensembles for quantum memories
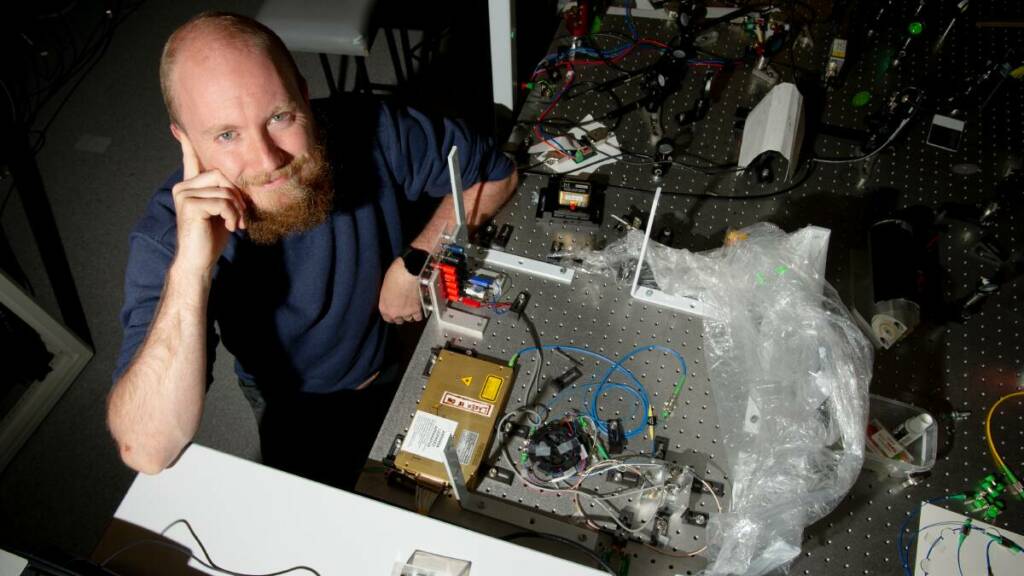
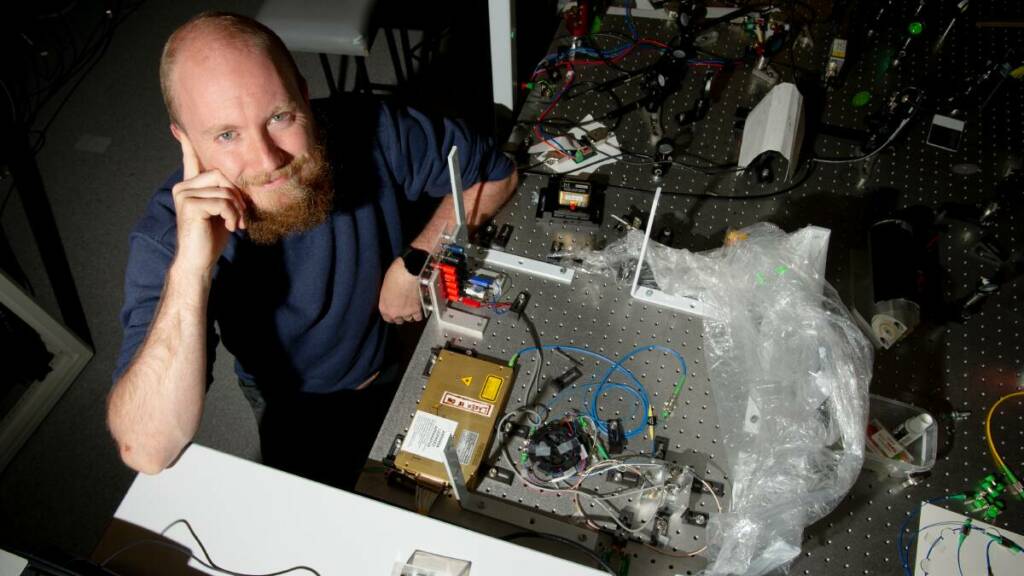
Date & heure
04/04/2018
Lieu
Jussieu, salle 210 couloir 13-23
Accueil
Quantum memories are integral to the realization of quantum information networks and quantum information processing. A promising platform is gradient echo memory (GEM) in cold atomic systems with demonstrated efficiencies of ~87%. We demonstrate the first application of a deep learning algorithm to a cold atomic system in order to increase the optical depth (OD) of our atomic trap and thus increase memory efficiency. We perform a 63 parameter optimisation and find solutions that are agnostic to considerations regarding monotonicity or continuity and vastly outperform human solutions increasing our optical depth by (81+-3)%. We also observe a physical change in the atomic cloud corresponding to the spatial distribution of the atomic ensemble and apply the optimisation to the GEM protocol.